The modern recruitment process falls short of ideal as it remains burdened with paperwork and administrative tasks that take up too much time, leaving little room for connecting with candidates and stakeholders.
These inefficiencies, affecting both in-house and agency recruiters, might seem like a given or an inherent shortcoming of talent acquisition processes.
However, the reality is that most of the challenges in modern recruitment processes can be solved by AI.
Yet, to achieve this, we need to stop thinking of artificial intelligence as merely an automation tool, and see beyond obvious use cases. Using AI to simply automate process steps and move candidates throughout the recruitment funnel means negating its transformative potential.
Artificial intelligence has the potential to completely reimagine hiring, taking it from AI-assisted to AI-led. This transformation can be achieved with the help of AI recruiters, agents that work alongside their human counterparts in full synergy. With this approach, the focus in the recruitment process shifts away from the process steps to the outcomes and impact it drives for the business.
So let’s see how the recruitment process is changing, in practice, once AI is added to the mix.
Recruitment before AI: Process-focused, driven by automation
To make it easier to understand the impact of artificial intelligence on the hiring process, let’s work with the stages of the recruitment process as we know them today.
However, keep in mind that the shift towards AI-led autonomous recruitment might mean that some of these steps will no longer be necessary, or will no longer have the same weight in the overall recruiting experience.
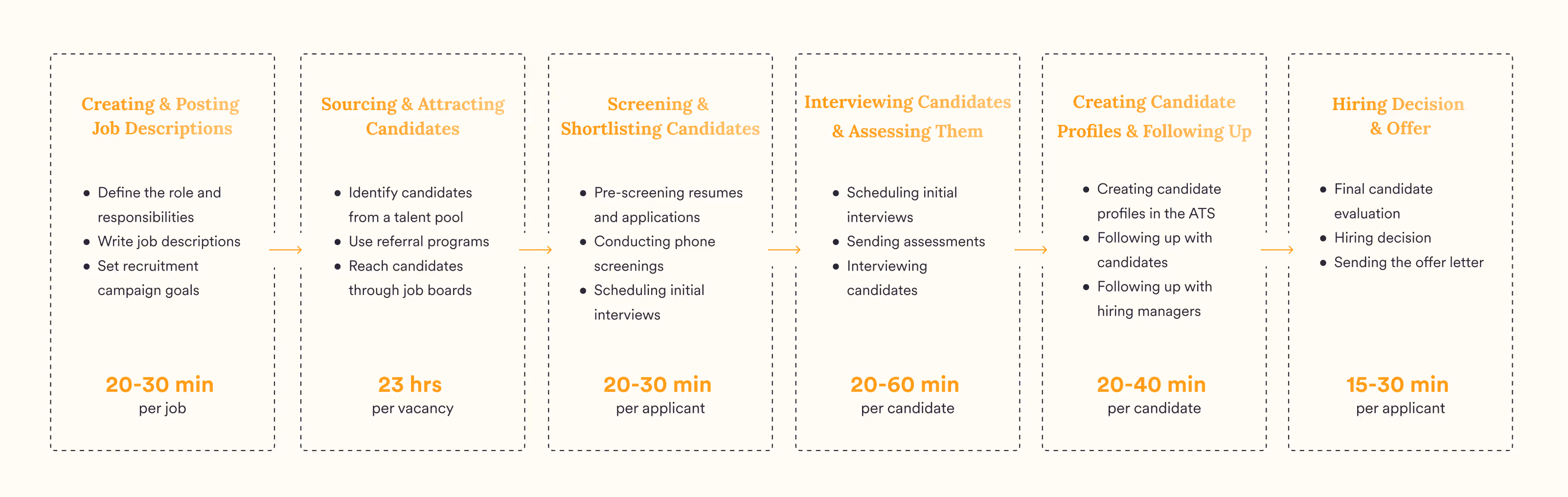
Sourcing & attracting talent
In traditional recruitment, even in heavily automated processes, this first step always requires human intervention. Typically, the search for quality candidates involves browsing through talent pools, social media platforms, and sharing vacancies across various job boards.
The talent acquisition team might use templates and automation tools to set up candidate outreach sequences, but this often ends up sabotaging their efforts, as candidates dismiss such approaches as spam. As outreach automation peaks, candidate engagement rates are expected to decline further.
On the sourcing side, recruiters depend on a mix of talent pools, memory, ATS data, and standalone Excel sheets. However, when talent pools are built by several team members and there’s little alignment between teams, and ATS data provides limited candidate details, finding suitable candidates becomes way more difficult than it should be.
To summarize, the main challenges in sourcing include:
- Inefficient search methods: Over-dependence on matching keywords can lead to inefficient talent discovery.
- Limited outreach precision: Traditional methods may not effectively target the most suitable candidates, leading to a broader yet less focused outreach.
- Time-consuming manual processes: The manual aspect of talent browsing and outreach can be time-intensive, reducing overall efficiency.
AI recruitment software can address these challenges, making sourcing fast and effective, and helping companies stand out in a crowded market, to attract the best candidates.
Assessing & screening candidates
In the modern approach to candidate screening and assessment, the administrative workload involved in the process leaves little room for extended engagement with the right candidates.
Especially for positions with a high volume of applications, recruiters swiftly skim through resumes, and assessments often adopt a one-size-fits-all approach, making it easy to overlook qualified candidates. Furthermore, due to the diverse range of candidates applying, recruiters frequently incorporate knock-out questions to filter out unsuitable applicants. Such questions are necessary but may not reveal much about the candidates.
.jpg)
Additionally, information stored in the applicant tracking system often emphasizes these procedural questions rather than focusing on the candidate's actual skills, potential, or personality.
So, to sum it up, the main challenges in screening include:
- Time-consuming manual processes: Steps like resume screening and candidate shortlisting take a significant amount of time, hampering the overall efficiency of the recruitment process.
- Prone to human bias: Subjective assessments are susceptible to unconscious bias, influencing decision-making and potentially impacting the fairness of the selection process.
- Limited scalability: Manual screening becomes increasingly challenging to scale, especially in high-volume recruitment scenarios, potentially leading to oversight of qualified candidates.
- Missed potential in unstructured data: Traditional screening methods may overlook valuable insights present in unstructured data, such as candidate portfolios, interviews, or project work, limiting the understanding of a candidate's capabilities.
In addressing these challenges, the integration of AI technologies promises to bring about transformative changes, optimizing efficiency, and promoting unbiased candidate evaluations.
Candidate interviewing and selection
The manual effort involved in the logistical aspects of scheduling interviews and communicating with candidates often consumes valuable time that could be better utilized in more strategic recruitment activities.
While automation has successfully addressed some of the challenges in the interview process, synchronizing calendars across teams and rescheduling no-shows remain persistent issues faced by both in-house and staffing recruiters. Also, the pre- and post-interview administrative tasks, ranging from intake calls with hiring managers to transcribing candidate interviews and writing candidate profiles, represent a significant time commitment.
.avif)
To summarize, the main challenges in interviewing include:
- Scheduling and syncing calendars: Repetitive tasks like scheduling interviews and coordinating calendars contribute to a significant time drain in current recruitment processes.
- Lack of standardization: In the absence of standardized criteria, current recruitment practices often lead to subjective and biased interviews, potentially filtering out top talent.
- Limited time for actual interviewing: Due to the time-consuming admin work before and after talks with job applicants, recruiters may struggle to conduct comprehensive interviews that delve into a candidate's true capabilities and potential.
While automation has brought notable improvements, the intricacies of scheduling and the need for standardized criteria underscore the importance of incorporating AI recruitment tools.
Decision-making & offer management
In the final stages of non-AI recruitment, decision-making and offer management encounter several challenges, mainly related to lengthy application processes that cause job seekers to withdraw, and to lack of data-driven insights when making the hiring decision.
Relying solely on candidate scoring lacks nuance, as scores do not account for a candidate's potential, feedback, and the assessment of transferable skills. While some tools aim to reduce subjectivity in scoring, when a recruiter passes a candidate to the hiring manager with only a score and no additional context, the hiring decision can become challenging and potentially flawed.
To sum it up, the main challenges in this phase include:
- Inconsistent criteria: Human recruiters may apply scoring criteria with variations. The absence of standardized benchmarks can result in divergent assessment approaches among recruiters, potentially introducing inconsistencies in candidate evaluations.
- Reliance on gut feeling: Without data-driven insights, the hiring decision often relies on human intuition, which may introduce potential bias. This reliance on gut feelings may lead to variations and inconsistencies in decision-making.
- Complex offer negotiations: The absence of data-driven insights into market benchmarks, candidate expectations, and budget constraints can hinder the optimization of offer management processes.
These hiring challenges collectively underscore the need for more streamlined, standardized, and data-informed approaches in the final stages of the recruitment process - and AI-powered tools can help.
AI-led recruitment: Impact-focused, driven by human-AI synergy
In an AI-driven reality, the recruitment process no longer needs to happen in a waterfall style, as AI is able to multitask, can execute tasks autonomously 24/7, and can work with both unstructured and structured data.
This means that the recruitment team can hand over most of the admin work to their AI counterparts, and focus on what they’re best at: Building relationships with candidates, hiring managers, and agency clients.
Although there are concerns regarding the replacement of human recruiters with AI technology, our belief is that artificial intelligence will be used as a tool to augment human decision-making, not replace it. Ethical implementation and focus on human-centric strategies are crucial for a successful AI-driven recruitment ecosystem.
With this in mind, here’s how implementing AI can transform each stage of the hiring process.
.avif)
Sourcing & attracting talent
In AI-driven recruitment, sourcing shifts from keyword-based searches to always-on predictive matching based on job descriptions and requirements, candidate skill set, personality, and culture fit.
AI recruiters or assistants navigate through talent pools 24/7, identifying ideal applicants and proactively engaging with potential candidates - and all of this happens with little to no human intervention.
The driver behind this always-on sourcing machine is the Talent Space, powered by graph databases.
Without making this too technical, imagine a social network where new connections are recommended based on similar interests, group belonging, and so on. Each person is a node in the network, and there’s no limit to the number of other “nodes” it can connect to. This is how networks like Facebook or LinkedIn work.
In an AI-powered Talent Space, the nodes are not only candidates, but also their CVs, social media interaction data, interview recordings, assessment results, hobbies, values, and so on. All these data nodes or talent mind maps can be scanned by AI in real time, and the data can be automatically matched with the job requirements coming from hiring managers.

At the same time, AI systems can debrief intake calls and launch targeted social media campaigns, reaching relevant applicants through their preferred communication channels, and engaging with them through contextually-enriched recruiting chatbots. The outreach messages can be highly personalized, as the AI can access all the information it finds when searching the Talent Space.
This results in more streamlined sourcing initiatives, more diverse and qualified candidate pools, less time doing admin work, and overall more effective talent acquisition.
We covered the topic of AI's impact on sourcing in the video below.
Candidate screening & assessment
AI can be used to streamline the screening and assessment phase too. Unlike non-AI screening, which works like a factory line, focusing on speed rather than candidate experience, AI-led recruiting gives the candidate the spotlight.
Among others, AI-driven screening uses automated resume parsing and context extraction, skills-based assessments with bias mitigation algorithms, and video interviews with AI-powered analysis for deeper insights.
Although this new way of working still relies on reading resumes, artificial intelligence systems can also go through candidate calls and video interviews, and can review the data collected from the Talent Space.
Moreover, AI can create personalized assessments on the go, to mitigate the potential risks it sees when evaluating a candidate’s profile. Instead of using only one data point and out-of-the-box assessments, AI can make the experience candidate-friendly, resulting in more flexibility and more focus on the candidate itself.
The screening can be done in the background, automatically, with candidates engaging in their own timing. This removes the randomness of standardized assessments, creating a truly candidate-focused hiring experience. The result is faster screening, fairer decisions, deeper insights into candidate potential.
%20(1).jpg)
Candidate interviewing and selection
In the interviewing phase, AI can be used to bring interview-related admin down to zero, freeing up recruiters so they can focus on candidates.
While automation technology is useful for scheduling and streamlining pre- and post-interview candidate communication, it often lacks a human touch. For example, templated outreach emails that invite candidate to interviews often lack personalization, and out-of-the-box candidate rejection emails often lack empathy.
Given that modern recruitment software can’t handle unstructured data, that’s where the automation stops.
Artificial intelligence doesn’t have this limitation. It can work with any type of data source - be it resumes, interviews, audio calls, or written messages.
- In the pre-interview phase, AI can listen to intake calls, review candidate resumes and profiles, and draft interview questions. This gives recruiters more context about candidates, and helps them enter interviews with confidence.
- During the interviews, AI can assist with real-time transcription, as well as with note-taking. Both these tasks are very time-consuming when done manually, and they often distract the recruiter from the interviewee. With the help of AI, recruiters can focus on candidates, instead of note taking and typing.
- Finally, post interview, AI recruiters - the assistants of human recruiters - can share interview summaries, highlight unanswered questions, or draft candidate profiles for the hiring managers. AI can ensure objective evaluations based on the candidate’s skills, experience and potential, and the actual role requirements.
As you can see, AI-powered interviewing software can automate these repetitive tasks, streamlining the workflow before, during, and after the interview. This gives recruiters their time back and improves both the candidate experience and the recruiter experience.
.avif)
Decision-making & offer management
Finally, AI can support in the decision-making process, by removing bias and subjectivity, and ensuring a fair assessment.
In staffing agencies and recruitment service providers, artificial intelligence can create commercial profiles for candidates, taking into consideration all the candidate data and context.
This can help hiring managers make better decisions - however, AI should never take over the actual hiring decision. This is the only step in the entire recruitment process, along with the interview, that we believe should still be handled by humans.
In the offer stage, AI can support with writing offer letters and negotiating with candidates, personalizing the communication to increase success rates.
Overall impact of AI-driven recruitment processes
As you can see from the above, AI-driven recruitment takes away all the administrative work, bringing admin down to zero, and freeing up TA teams so they can focus on building relationships with candidates and clients and shaping recruitment strategies to drive business outcomes.
%20(1).png)
This has impact on both stakeholders and recruitment metrics.
Impact on stakeholders
- Hiring managers: Improved efficiency, better hires, data-driven decision-making.
- Recruiters: Time-saving automation, deeper candidate insights, focus on strategic tasks.
- Candidates: Personalized experience, fairer selection process, faster feedback.
- Organizations: Higher ROI, stronger employer brand, improved talent retention.
Impact on recruitment metrics
- Efficiency: AI takes over admin tasks. Time to fill and time to hire metrics improve.
- Effectiveness: Data-driven insights lead to better talent selection and improved talent management. Candidate conversion rates, quality of hire, and retention rates improve.
- ROI: For staffing agencies and recruitment service providers, AI-led recruitment means faster placements and thus faster return on investment.
- Fairness: AI tools can mitigate bias and promote inclusive hiring practices. Candidate selection is no longer affected by subjective opinions.
- Candidate experience: Personalized interactions and faster communication create a positive candidate experience. Candidate satisfaction scores and employer ratings improve.
To conclude, artificial intelligence can reshape recruitment and act as a catalyst for reimagining the entire hiring landscape. AI goes beyond streamlining process steps; it has the power to shift recruitment from being AI-assisted to AI-led.
The key lies in adopting AI agents that can work with unstructured data, and in treating these AI recruiters as helpful workmates - intelligent agents working seamlessly alongside their human counterparts.
This collaborative synergy allows for a holistic transformation where the focus shifts from the intricacies of process steps to the broader outcomes and impact on the business.
